- By Eduardo Gallestey, PhD, Luis Domínguez, PhD, Simo Saynevirta, S. Mohan Kumar, PhD
- Process Automation
Summary
Fast Forward
- Optimizing processes to be efficient and competitive can be accomplished across all systems with IoT and cloud computing"-based independent APC solutions.
- New platform-independent APC provides the core functionalities of APC traditionally integrated in DCS systems with calculation engines and data communication run as independent processes.
- Configurations may include industrial communication standards, such as OPC, to connect users and apply APC with any system.
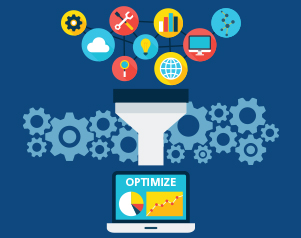
Industrial information technologies take process optimization to the next level
By Luis Domínguez, PhD; S. Mohan Kumar, PhD; Eduardo Gallestey, PhD; and Simo Saynevirta
APC technology makes use of model predictive control and moving horizon estimation strategies. These advanced control and state estimation strategies have been widely studied and improved over the years. They make use of a linear or nonlinear mathematical model of the plant and powerful optimization algorithms to estimate unmeasured states and control process variables. The result of applying such strategies is optimal control policies that drive the plant to desired targets.
The benefits of APC technology are clear: the opportunity to improve process operation, minimizing variation of key variables while considering process constraints. These operational benefits translate into financial ones due to increased throughput and minimized energy use. When integrated with distributed control systems (DCSs), APC technology gives users the ability to take advantage, among other things, of the system's distributed resource allocation, redundancy, and communication, as well as the built-in cybersecurity infrastructure.
Recently companies have developed new platform-that is, DCS-independent APC. These provide the core functionalities of APCs traditionally integrated in DCS systems, with the difference that the calculation engines and data communication and exchange run as independent processes.
A platform-independent APC is made up of off-line and online components (figure 1) that make the development and deployment of advanced controllers a fully streamlined process:
- APC model builder (off-line)
- APC configuration manager (online)
- APC run-time engine (online)
- APC manager service (online)
APC model builder
Process modeling and controller design tasks are carried out in an APC model builder, a client application that allows authorized users (e.g., control and commissioning engineers) to create, test, and deploy process models, soft sensors (i.e., analytics), and advanced controllers.
Authorization to generate valid soft sensors and controllers for deployment is handled through APC certificates, electronic documents that ensure the authenticity and integrity of controllers and soft sensors. When exporting a controller or soft sensor (an XML file deployable in the APC configuration manager, see below)\, an APC model builder will digitally sign a controller or soft sensor only if a valid certificate associated with the authorized user is found.
The user also has the possibility of importing large data sets and performing advanced data operations and analytics, such as resampling, interpolation, or filtering, as well as performing open- and closed-loop simulations on the imported data.
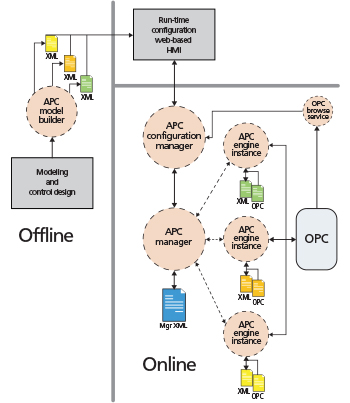
Figure 1. Platform (DCS) independent APC architecture. This example APC model builder uses cloud infrastructure providers, such as Microsoft, to leverage their encryption and cybersecurity technology for an added layer of protection to data and communication. Online functions must reside at the plant site, while other functions can be at the local site or in cloud applications.
APC configuration manager, run-time engine, and manager service
The configuration, deployment, and monitoring of advanced controllers is carried out in an APC configuration manager, a web application that allows remote users to create, delete, edit, import, and export advanced controllers. Once imported, an APC configuration manager verifies the signature of the controller file. If an APC configuration manager cannot find a valid certificate, or the signature is invalid, the controller file is rejected, and an error message is displayed.
APC configuration manager implementations can include industrial communication standards, such as OPC, to allow users to connect to the plant, create or configure existing tags, and map them to variables and parameters of imported controllers.
Data exchanged between the plant and the controller is typically secured with authentication and encryption using high-security standards, such as secure web socket communications (HTTPS), certificate-based security (SSL), and Transport Layer Security (TLS).
Once advanced controllers are successfully loaded, an APC configuration manager creates instances of the APC run-time engine, a service application containing the optimization algorithms that runs in the background and carries out the computation.
Each APC run-time engine instance is monitored by an APC manager service, a dedicated process that ensures each APC run-time engine instance is available and in operation. In the event of engine failures, the APC manager service restores the failed instance within the sampling period of the assigned controller to ensure continuous availability.
The APC manager service is also responsible for routing and displaying events and messages associated with specific APC run-time instances. Any alarm and event with its severity previously configured in APC model builder is also displayed in the web application by the APC manager service. In essence, the APC manager service and the APC run-time engine work synchronously to ensure the robust operation of deployed APC controllers.
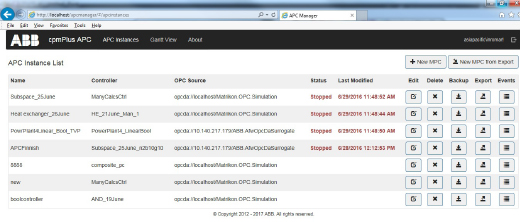
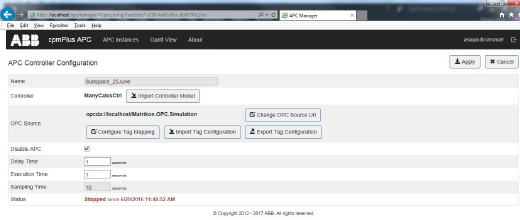
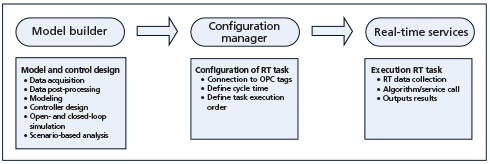
Figure 4. Streamlined and merged workflows and technologies involved in the deployment of advanced process controllers
IoT platform integration
Platform-independent APC can also be integrated into IoT platforms, offering the possibility to make automated operational decisions (e.g., control and optimize processes and equipment) from an industrial cloud. Communication from the controller to the plant is accomplished via the OPC UA, an IoT-enabled protocol, implemented in the APC configuration manager. IoT platforms offer additional cybersecurity, communication, visualization, and memory infrastructure-critical for the execution of computing-intensive applications, such as advanced process control and optimization.
By using cloud infrastructure and services, such as Microsoft Azure or Amazon Cloud, deployed control and optimization applications gain further protection against intrusion, penetration, induced anomalies, and distributed denial-of-service attacks, among other threats.
IoT platforms also incorporate the latest visualization technologies, such as HTML5, offering great flexibility for human-machine interface designs. By utilizing user interface and user experience templates integrated into IoT platforms, the control/commissioning engineer can develop straightforward interfaces for tuning and configuring the parameters of deployed controllers (figure 5)\, as well as visualizing the data of process variables (figure 6).
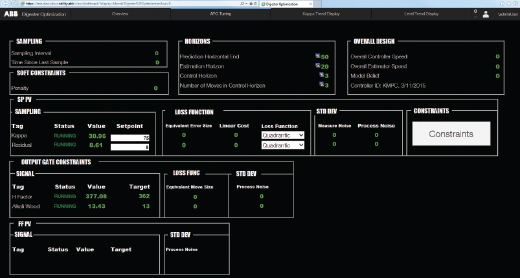
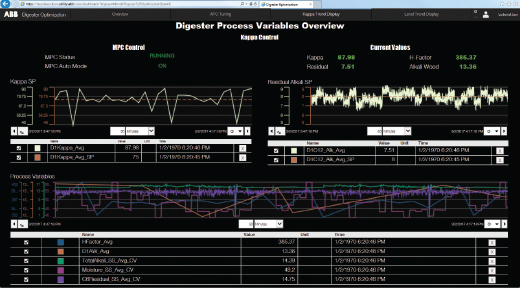
Improvements in modeling
Along with IoT integration and improvements in data communication and visualization, platform-independent APC technologies continue to be extended with additional data-driven models, soft sensors, and analytics, as well as new graphical modeling blocks.
Soft sensors and analytics
Soft sensors are models that can be used to infer nonexistent or expensive measurements, increase the frequency, or provide backup for nonreliable measurements. They can be derived from first principles or process data and can be deployed directly to the APC run-time engine system. There are various types of soft sensors that can be used in APC model builder:
- Graphical (i.e., first-principle)
- Linear regression-based
- Nonlinear regression-based
- Principal component analysis (PCA) regression-based
- Artificial neural network (ANN) regression-based
Graphical soft sensors can be used when a first-principle model (derived from knowledge or experience) of a process variable is available. For example, a nonmeasured temperature at the exit of a tubular reactor may be inferred from two or more sensed measurements at the beginning and middle of the reactor. When no knowledge of the relationship between variables is available, the user may utilize previous measurements to fit a model to the data. If the relationship among sensed variables is linear, a linear or PCA regression-based soft-sensor model may be used to infer the unmeasured variable. On the other hand, if the relationship among sensed variables is suspected to be nonlinear, a nonlinear or ANN regression-based soft sensor model may be used. The user can train and test multiple models to identify the model with the best performance statistics or model fitness.
Use case: Prediction of unmeasured variables in a pulp and paper application
One example where an ANN regression-based soft-sensor model has been used is to infer and control the kappa number, a quality measure of the lignin level inside continuous digesters in the pulp and paper (P&P) process. This number is difficult to measure and control due to the lack of sensors in digestive zones and different physical characteristics of the fed chips. By using available measurement data of process variables at the digester's blow line, such as H factor, total alkalinity, moisture, and residual alkalinity, an ANN soft sensor model (trained and validated offline, figure 7) predicts the kappa value inside the digester. This is then used by the controller.
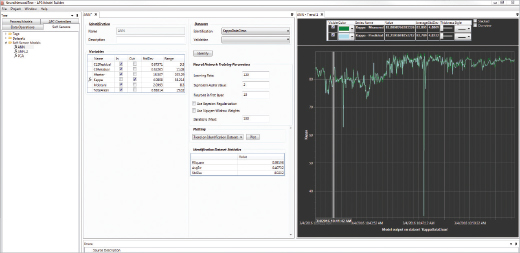
Figure 7. ANN regression-based soft-sensor model identified, trained, and validated in an APC model builder using collected data of a P&P process. The identification tab displays the input and output variables as well as the network tuning parameters used to identify the soft-sensor model. The tab displays a validation plot of the predicted model output versus the variable’s collected data.
Mixed-logical models
In addition to linear and nonlinear models, the new versions of APC model builders now support mixed-logical models (implemented using Boolean inputs, outputs, and blocks together with linear and nonlinear blocks). This feature allows the user to model explicitly hybrid dynamical systems, such as piecewise affine, discrete hybrid automation, and switched affine systems.
Use case: Optimization of power generation inside ships
An example where this feature has been used is in the optimization of the generated power inside vessels. It allows load balancing inside ships, modeling, and optimization of switching (on/off) decisions of the ship's diesel generators in the face of varying power demands. Using the multi-objective feature and a model of the specific oil consumption, a measure of fuel efficiency, the APC controller determines the optimal load distribution among four diesel generations (including which generators to keep switched on or off). It does this while minimizing the overall fuel consumption.
Figure 8. A graphical model of a power plant with four diesel generators inside ships developed inside an APC model builder. The desktop canvas in the first tab displays the graphical model; whereas the next tab displays the open-loop simulation plots of the model.
Gain delay models
In addition to the data-driven models, such as subspace and transfer functions, the new versions of APC model builders now support the identification of gain delay models. These are straightforward models that can explain data well with few parameters and are used extensively in practice.
The gain delay model consists of a multiple-input, multiple-output state space model, identified by combining multiple-input, single-output transfer functions containing either pure delays (zero order dynamics) or time constants (first order dynamics) of each output. For cases where the identified model contains many states, the user can obtain a compact model by applying a model reduction step using various available methods.
Use case: Optimization of ventilation systems in underground mines
One case where gain delay models have been used successfully is in the optimization of ventilation systems in underground mines. Mine ventilation is critical for maintaining an adequate air flow level, climate control, and quick evacuation of blast gases. Due to the scale and complexity of the layout, a number of large fans must be strategically distributed throughout the mine (figure 9). The suboptimal operation of such fans can lead to major increases in total energy consumption.
Using collected data from step tests on key process variables of a mine's ventilation systems (e.g., fan speed, cell flow response, differential pressure, and power consumption), gain delay models are identified and used for the control and optimization of the total power consumption of fan networks. The implementation of developed advanced controllers in the distributed control system of mines often results in energy savings of 30-50 percent per year.
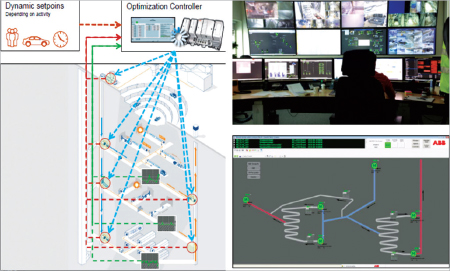
Figure 9. The layout of a mine whose ventilation system has been optimized using a gain delay model identified in an APC model builder.
Figure 10. Identification of a gain delay model in an APC model builder. The user can tune the parameters to arrive at a model that explains the data with sufficient accuracy.
The tab to the right displays the validation plots of the model (data versus model prediction).
Time-varying parameters
Time-varying parameters (TVPs) allow the possibility of dynamically incorporating changing parameters to process models, controllers, or soft sensors. This feature is suitable for developing advanced controllers with time-varying constraints. For example, upper or lower bounds in input and output variables can be dynamically adjusted over a given horizon to accommodate a more flexible or stricter operation. Another possibility is for forecast tracking. By configuring a set point in the controller as TVP, the controller automatically follows a given forecast, which may be provided by a forecasting system.
Estimated parameter and Boolean blocks
The new versions of APC model builders come with estimated parameter blocks that can be used for estimating unknown parameters of a model; whereas Boolean blocks can be used to build mixed logical models.
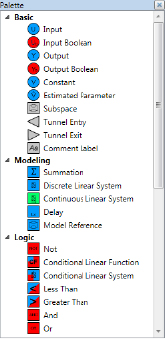
Figure 12. Estimation of fouling parameters in a heat exchanger using an APC model builder
Use case: Estimation of heat transfer coefficients in heat exchangers
An application where the estimated parameter block is useful is real-time performance monitoring of heat exchangers in the oil and gas industry. In heat exchangers, fouling can occur due to the deposition and accumulation of materials, such as algae or scale on the internal or external surfaces. These phenomena often lead to a significant drop in operational efficiency. Estimating the value of heat transfer coefficients allows process engineers to decide on a maintenance activity that can improve the heat transfer and thus the efficiency of the heat exchanger. When implemented online, the real-time estimation of the heat transfer coefficients allows the control system to implement an optimal APC model builder.
APC technology continues to evolve for many in the industry. Improved design and modern user interface modeling platforms facilitate data analysis and greatly reduce modeling effort. This offers users a greater flexibility in evaluating and testing models that best fit process data. This approach enables accelerated commissioning and online monitoring of advanced controllers.
Thanks to the technological advances brought by IoT, it is now possible to deploy and monitor advanced controllers to and from an industrial cloud. This offers the possibility of new operational software-as-a-service business models and collaborative operations for multisite optimization.
Reader Feedback
We want to hear from you! Please send us your comments and questions about this topic to InTechmagazine@isa.org.